.
.
Mastering Data Quality
Best Practices for Product Data Governance and Successful Pricing Initiatives
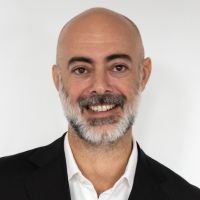
Author
Abstract
Introduction to Pricing Initiative Challenges
Pricing initiatives can be derailed by a lack of executive commitment, resistance to change, or lack of resources or investment... the list is long, and anyone involved in implementing pricing projects is familiar with most of them.
However, even within these undesirable scenarios, you can achieve some level of success. For example, if there is no executive buy-in or your budget is very limited, you can always run a small pilot with one business unit and prove the impact.
But there is one limiting factor in any pricing or revenue management project: data quality.
No matter how good your model is, if you are using machine learning or any other type of artificial intelligence, the adage "garbage in, garbage out" always applies.
Pricing models are fed with many different data sets: transactions, customers, competitors, products, and weather forecasts...
During a pricing conference, I conducted a survey and product data was a headache for 71% of the audience, so in this article, we will cover some best practices for product data governance that can be adapted to other contexts.
In most cases, brick-and-mortar companies are lagging in digitalisation, and the focus is on other parts of the business, mostly on ensuring smooth transactions. On top of that, acquisitions and integrations are part of the growth path, so we have the perfect breeding ground for a perfect product data mess.
Mastering Data Quality: Ensuring Consistency and Quality in Pricing Models
In today's increasingly data-driven business landscape, product data consistency is crucial for organisations to maximise the potential of their data and make informed decisions. Ensuring that all departments and business units are on the same page when it comes to product data can be a challenging task, but it is essential for driving growth and maintaining a competitive edge.
- The Importance of Product Data Consistency
The entire company needs to speak the same language when it comes to product data. Regardless of the business unit, geographic region, or department, a part must always have the same SKU ID. Period. Sounds basic, right? Go and check in your company. You might be surprised.
Similarly, SKU aggregations to build meaningful and relevant hierarchies must be the same, as well as units of measure and conversions between different units.
I remember one sales organisation that decided to classify 25-yard rolls as 22 meters instead of 22.86 meters because it was "easier to manage in the warehouse", so imagine how tricky to answer the simple question “How many meters have you sold during the last 12 months?”
- Key Requirements for Product Data Excellence
Based on personal experience and successful company practices, three basic requirements can be identified for product data excellence:
- A dedicated team responsible for data governance and feeding data into all enterprise systems.
- Clear (and strict) rules for product classification and product data attributes.
- Processes in place for regular cleansing and maintenance to keep data content and structure consistent. - Challenges in Decentralized Organizations
We see pretty often in decentralised organisations, or with very powerful business units, or in companies that grow and extend via acquisitions, that product data is managed at a local level, in siloes where each subsidiary does its best but without a common strategy and processes.
In these scenarios, data quality can be okay-ish -not quite often, to be frank, but consistency is hard to see.
- Building Consistency Across Business Units: Inclusive Approach to Product Data Governance
Consistency or, in other words, that everybody talks the same language when it comes to product attributes and aggregation is capital to:
- Take advantage of larger datasets that are required to feed segmentation and classification models, and
- Build meaningful and actionable reports, especially when consolidating information from different areas of the business.
To build consistency, you need to establish some common ground and also acknowledge that, whatever you create, you are not going to satisfy everybody.
But this does not mean you have to establish your product data requirement and processes in a “data lab” and cascade it down.
The best practice here is to collect requirements and inputs from corporate but also deep-dive and make sure local organisations and business units are part of the process.
It is not always the case, but most of the time, there are good reasons why people do stuff in a certain way. But that a certain product aggregation makes much sense in, let’s say, France due to local market specificity; it doesn’t mean it can work in Australia or Belgium as well.
The best approach here is to listen to everybody who can contribute, gather best practices, ideas, and requirements, identify the highest common denominators, make a case for why and how the initiative will help the company achieve its goals, and explain to all parties what is in it for them. - Leveraging Top Management Support for Successful Implementation
Persuasion, good communication skills and making everybody feel part of the process are your allies, but notice that from time to time, or with some individuals or departments, you will need reinforcement and support from the top management to make sure they embrace changes, so make sure you will have this support from top executives within your company.
Implementing Product Data Governance: Centralization, Cleansing, and Scope Management
In the world of business, data is king. However, having access to data is not enough to drive growth and success. It's what you do with the data that matters. That's where a product data "dictionary" and "grammar" come in. But once you have built this foundation, what comes next?
- Establishing Product Data Governance Roles and Building Your Team
Once you have built your product data "dictionary" and "grammar," it's time to determine who will be responsible for making decisions related to product data and who will be responsible for introducing the data into the system. There are different approaches to this, but one that has demonstrated success is to centralise product data governance to maintain consistency and efficiency in data feeding. As a result, you should prepare to ask for the necessary budget and create a team to handle these responsibilities, which will need proper training. - Implementing Robust Data Cleansing Processes and Monitoring Quality Metrics
Once you know how and who manages your product data, you also need to put robust cleansing processes in place working at two levels: quality and legacy.
No matter how well you have defined your rules of engagement to classify and define product attributes, and no matter how well the team is trained, there will always be mistakes. That’s a fact, and I have seen pricing initiatives derailing after a promising start a few times due to product data quality issues that generate a lack of trust or even the impossibility of measuring and tracking the impact.
You need to define data quality metrics to track consistency and allocate time to regularly spot-check.
The rule “it should be OK as nobody is complaining” does not work here.
Basic data quality analysis should ensure that:
- Each SKU is assigned to one and only one classification string,
- Data attributes population follow a normal distribution, and
- Data attributes outliers and those sitting in the long tails (above 22- in absolute value) are reviewed and fixed. - Managing Legacy Data and Defining the Scope for Prioritizing Product Data:
Legacy data management and cleansing is a large and complex topic that will require another article to just scratch its surface, so let’s cover the most basic principle: defining the scope. You can’t fix everything in one go.
You might need to start with some specific product ranges or business lines, but if this is not given, common sense is always a good starting point.
Identify the 20% of your products that generated 80% of your sales during the last 24 months so you can work with the current year and compare with the previous one, and then make sure all those products follow everywhere in the “dictionary” and the “grammar” you have already established.
From that, expand the scope by adding more products in waves until you cover 99% of your revenue for the last five years.
The Final Principle: Data First, Then Pricing
One final concept that should be carved in stone and is valid for all companies, all industries and all kinds of pricing or revenue management initiatives is that one:
Only start your pricing project if you have got good data.
If not, fix it first.
Not the other way around, not in parallel.
First, data, then pricing.
Conclusion
In conclusion, the success of any pricing initiative is highly dependent on the quality of data fed into the models. By establishing transparent processes and rules for data governance, ensuring consistency, and emphasising the importance of data quality, businesses can overcome the challenges associated with data management and move forward with effective pricing initiatives. Always remember: data quality comes first, and pricing initiatives can only follow once the foundation of reliable data has been established.
By implementing these strategies, businesses can unlock the true value of their data and set themselves up for long-term success.